Student(s): Marco Kemmerling;
Supervisor(s): Dr. Rachel Cavill;
Year: 2016;
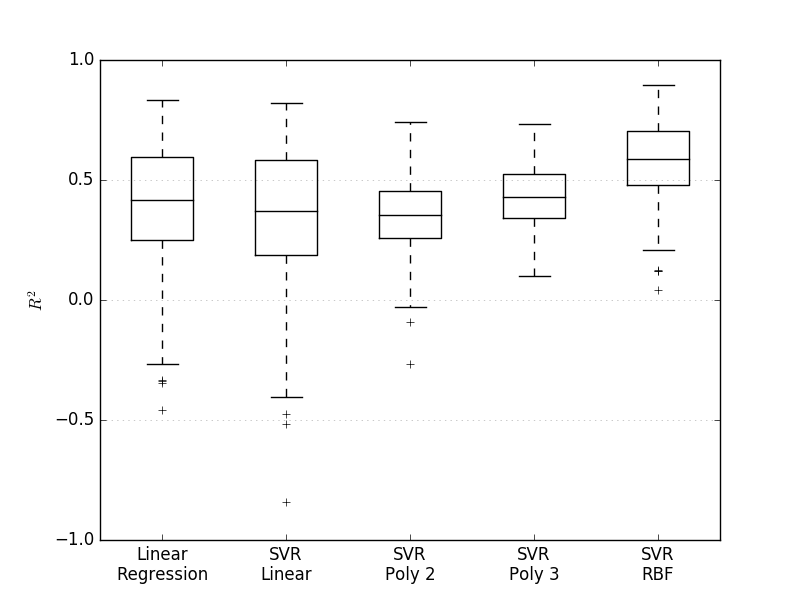
Problem statement and motivation:
The relationship between protein levels and other biological measures like gene or microRNA expression is not very well understood. To further the understanding of this area, it would be useful to find connections between protein expression and other possible measurements, which could be a first step towards the development of a model describing these relationships.
To approach this task, I will use machine learning techniques to predict protein levels in cells based on other available information like gene, protein or microRNA expression.
Research questions/hypotheses:
In the present project we aimed at answering the following research questions:
- Which (combination of) features are relevant to the prediction task?
- Which machine learning techniques yield the best results?
- Can the same features be used to predict any protein?
- What is the impact of including more/less datasets?
Main outcomes:
- Linear regression and support vector regression were examined as methods to predict protein levels. Kernels used for SVR were linear, polynomial of 2nd and 3rd degree as well as radial basis function. The best performing technique turned out to be support vector regression with a radial basis function kernel.
- Applying feature selection prior to linear regression significantly improved performance. Feature selection via the Lasso elevated performances to nearly the same level as SVR with the RBF kernel. Univariate feature selection and recursive feature elimination were tested as well, but did not result in the same performance increase as applying the Lasso. Feature selection with SVR proved to be unsuccessful.
- Features were mainly comprised of (other) protein expressions. Overall, including gene data did not prove to be very useful, although only adding the gene coding for the protein in question did lead to a small but statistically significant improvement.
- A different set of features is relevant for each protein. However, some features are useful for predicting the majority of all proteins, while others are only useful for a small subset of predictions.
- Datasets from different tissues/cancer types were not directly compatible with each other. However, useful models could be built on a combination of datasets from different sources.